Original Equipment Manufacturers (OEMs) are central to the industrial ecosystem, supplying crucial components and products across industries. Effective inventory management is vital for ensuring the availability of spare parts and critical components, which is essential for maintaining high fill rates and reducing downtime. Traditional methods often lead to inefficiencies such as overstocking and stockouts, impacting the supply chain and profits. Research by Aberdeen Group shows that poor inventory practices can cut potential profit by about 30%.
Advanced solutions like AI and Machine Learning (ML) offer significant improvements. These technologies enable OEMs to achieve unprecedented levels of efficiency and accuracy in inventory management. AQe Digital provides digital solutions that use AI/ML to enhance inventory management efficiency by 30-35%.
Challenges Faced by OEMs Without AI/ML Integrated Inventory Management
OEMs that rely on traditional inventory management systems face a range of challenges that can hinder their operational efficiency and profitability. Without the integration of AI/ML, these challenges can become even more pronounced, leading to significant operational disruptions. Below are some common issues that OEMs encounter without AI/ML integration:
1. Inaccurate Demand Forecasting
One of the most significant challenges that OEMs face is inaccurate demand forecasting. Traditional inventory management systems often rely on historical data and simple algorithms that may not account for complex variables such as market trends, seasonal fluctuations, and external factors like economic conditions or geopolitical events. As a result, OEMs may struggle to predict future demand accurately.
Inadequate demand forecasting can lead to two major problems: stockouts and overstocking. Stockouts occur when a company fails to maintain sufficient inventory levels to meet demand, leading to lost sales, customer dissatisfaction, and potential damage to the brand’s reputation. On the other hand, overstocking results in excess inventory that ties up capital and increases holding costs, such as storage, insurance, and depreciation.
Moreover, without AI/ML, OEMs may miss emerging market trends and seasonal patterns that could influence demand. For example, a sudden increase in demand for a particular component due to a new product launch or a shift in consumer preferences might go unnoticed, leading to poor inventory planning and missed sales opportunities.
2. Human Errors
Human errors are another major challenge in traditional inventory management systems. Manual processes, such as data entry, stock counting, and order processing, are prone to mistakes, which can lead to significant inventory discrepancies. Studies have shown that manual inventory processes can cause up to 70-80% of inventory discrepancies, resulting in inefficiencies that can ripple through the entire supply chain.
These errors can have a range of negative consequences, including incorrect inventory levels, misplaced items, and inaccurate reporting. Such inaccuracies can lead to production delays, missed delivery deadlines, and increased operational costs. In a worst-case scenario, these errors can disrupt the entire supply chain, leading to lost sales, customer dissatisfaction, and damage to the company’s reputation.
3. Increased Lead Times
Lead times—the time it takes from placing an order to receiving the goods—are a critical factor in inventory management. Longer lead times can disrupt production schedules, delay order fulfillment, and increase the risk of stockouts. Traditional inventory management systems often lack the real-time data-sharing capabilities needed to reduce lead times effectively.
Without AI/ML integration, OEMs may face challenges in collaborating with suppliers, leading to extended lead times. For example, a lack of real-time visibility into inventory levels and supplier capabilities can result in delayed shipments, missed deadlines, and increased operational costs. In a competitive market, these delays can have a significant impact on customer satisfaction and loyalty.
AI/ML integration, on the other hand, enables real-time data sharing and collaboration with suppliers, improving lead times by 10-15%. By leveraging AI/ML technologies, OEMs can monitor supplier performance, anticipate potential delays, and take proactive measures to mitigate risks. This level of visibility and control can significantly reduce lead times, enhance production planning, and improve overall supply chain efficiency.
4. Inefficient Production Planning
Effective production planning requires accurate and up-to-date inventory data. Without real-time inventory visibility, production planning becomes a guessing game, leading to delays or disruptions in the manufacturing process. For example, if an OEM is unaware of a shortage in a critical component, production may be halted, leading to missed deadlines and increased costs.
In traditional systems, production planning is often based on outdated or inaccurate data, resulting in inefficiencies that can ripple through the entire supply chain. For example, overproduction of certain items may lead to excess inventory, while underproduction may result in stockouts and missed sales opportunities. Without AI/ML, OEMs may struggle to align production schedules with actual demand, leading to suboptimal resource allocation and increased operational costs.
AI/ML integration can transform production planning by providing real-time insights into inventory levels, demand forecasts, and production capacity. By leveraging AI/ML technologies, OEMs can optimize production schedules, reduce lead times, and minimize disruptions. This level of precision and control can significantly improve operational efficiency, reduce costs, and enhance customer satisfaction.
5. Lack of Advanced Analytics
Traditional inventory management systems often lack advanced data analytics capabilities, which are crucial for identifying patterns and trends in inventory data. Without AI/ML, OEMs may rely on reactive decision-making, addressing issues as they arise rather than proactively planning for future challenges.
For example, traditional systems may not be able to identify seasonal trends, customer preferences, or emerging market opportunities. As a result, OEMs may miss out on critical insights that could inform strategic decision-making and drive business growth. Without advanced analytics, OEMs may struggle to optimize inventory levels, forecast demand accurately, and respond to changing market conditions.
AI/ML integration, on the other hand, provides OEMs with powerful data analytics tools that can analyze large volumes of data quickly and accurately. These tools can identify patterns, trends, and anomalies in inventory data, enabling OEMs to make informed decisions and optimize inventory management. By leveraging AI/ML technologies, OEMs can move from reactive to proactive decision-making, reducing risks and enhancing operational efficiency.
6. Poor Decision-Making
Effective decision-making is critical to successful inventory management. However, without AI/ML-driven insights, OEMs may struggle to make informed decisions that align with their strategic goals. Traditional systems often provide limited visibility into inventory data, making it difficult for OEMs to assess the impact of different scenarios on their inventory levels, production schedules, and supply chain operations.
For example, without AI/ML, OEMs may struggle to assess the impact of a sudden increase in demand, a supplier disruption, or a change in market conditions. This lack of visibility and control can lead to poor decision-making, resulting in suboptimal inventory levels, increased costs, and missed sales opportunities.
AI/ML integration provides OEMs with real-time insights and predictive analytics that can enhance decision-making and improve scenario planning. By leveraging AI/ML technologies, OEMs can simulate different scenarios, assess their impact on inventory levels and supply chain operations, and make informed decisions that align with their strategic goals. This level of precision and control can significantly improve inventory management, reduce risks, and drive business growth.
How Our AI/ML Services Can Streamline Inventory Management by 30-35% for OEMs
AQe Digital’s AI/ML integration services offer a comprehensive solution for enhancing various aspects of inventory management, from real-time data analysis to automated decision-making. These technologies are designed to process large volumes of data quickly and accurately, enabling OEMs to optimize their inventory management practices and achieve significant improvements in efficiency and accuracy.
1. Improved Inventory Tracking (40-50%)
Inventory tracking is a critical component of effective inventory management. Traditional systems often rely on manual processes or basic software tools that can result in inaccuracies, inefficiencies, and delays. By integrating AI/ML technologies, OEMs can significantly improve their inventory tracking capabilities, leading to enhanced operational efficiency and reduced costs.
- Data Collection and Analysis: AI/ML services gather and process data from various sources, including warehouse management systems, eCommerce platforms, and supply chain management tools. This data is then analyzed in real-time, providing OEMs with accurate and up-to-date snapshots of their inventory levels. For example, AI/ML algorithms can track the movement of goods within a warehouse, monitor stock levels across multiple locations, and identify discrepancies between physical and recorded inventory.
- Predictive Capabilities: AI/ML technologies go beyond simple data collection and analysis by offering predictive capabilities that can help OEMs anticipate future demand and optimize their inventory levels. For example, AI/ML algorithms can analyze historical sales data, market trends, and seasonal patterns to forecast demand for specific products or components. This level of foresight allows OEMs to proactively manage their inventory, reducing the risk of stockouts and overstocking.
- Automation: One of the key benefits of AI/ML integration is the ability to automate inventory tracking processes. AI/ML models can be developed to automate tasks such as stock counting, order processing, and inventory reconciliation, reducing the need for manual intervention and minimizing the risk of human error. For example, AI/ML algorithms can automatically update inventory records in real-time as goods are received, stored, or shipped, ensuring that inventory data is always accurate and up to date.
- Cost Reduction: By improving inventory tracking, OEMs can achieve significant cost reductions, particularly in areas such as storage, handling, and transportation. For example, AI/ML technologies can optimize the placement of goods within a warehouse, reducing the time and labor required for picking and packing. Additionally, AI/ML algorithms can identify slow-moving or obsolete inventory, allowing OEMs to take corrective action and minimize holding costs.
2. Optimized Demand Forecasting (50%)
Accurate demand forecasting is essential for effective inventory management. Traditional methods of demand forecasting often rely on historical sales data and basic statistical models, which may not account for complex variables such as market trends, seasonal fluctuations, and external factors. AI/ML technologies offer a more sophisticated approach to demand forecasting, enabling OEMs to achieve greater accuracy and precision.
- Advanced Algorithms: AI/ML algorithms can analyze vast amounts of data from various sources, including historical sales data, market trends, and external factors such as economic conditions or geopolitical events. These algorithms can identify patterns and trends that may not be apparent using traditional methods, providing OEMs with more accurate and reliable demand forecasts.
- Real-Time Adjustments: One of the key advantages of AI/ML-based demand forecasting is the ability to make real-time adjustments based on changing market conditions. For example, if a sudden increase in demand is detected, AI/ML algorithms can automatically adjust inventory levels and production schedules to ensure that stockouts are avoided. This level of flexibility and responsiveness is essential in today’s fast-paced and competitive market environment.
- Scenario Planning: AI/ML technologies also enable OEMs to perform scenario planning, which involves simulating different scenarios and assessing their impact on inventory levels and supply chain operations. For example, OEMs can simulate the impact of a sudden increase in demand, a supplier disruption, or a change in market conditions on their inventory levels and production schedules. This level of foresight allows OEMs to make informed decisions and proactively manage their inventory.
- Risk Mitigation: By optimizing demand forecasting, AI/ML technologies can help OEMs mitigate risks associated with stockouts, overstocking, and other inventory-related issues. For example, AI/ML algorithms can identify potential risks in the supply chain, such as supplier delays or quality issues, and recommend corrective actions to mitigate these risks. This level of control and visibility can significantly reduce the risk of operational disruptions and improve overall supply chain efficiency.
3. Proactive Maintenance (40%)
Proactive maintenance is a critical component of effective inventory management, particularly for OEMs that rely on complex machinery and equipment to produce their products. Traditional maintenance approaches, which are often reactive, can lead to unplanned downtime, production delays, and increased operational costs. AI/ML technologies offer a more proactive approach to maintenance, enabling OEMs to optimize their maintenance processes and achieve significant improvements in efficiency and cost savings.
- Condition Monitoring: AI/ML algorithms can be used to monitor the condition of machinery and equipment in real-time, identifying potential issues before they lead to equipment failure. For example, AI/ML algorithms can analyze data from sensors and other monitoring devices to detect signs of wear and tear, overheating, or other issues that could lead to equipment failure. This level of monitoring allows OEMs to take corrective action before a failure occurs, reducing the risk of unplanned downtime and production delays.
- Predictive Maintenance: In addition to condition monitoring, AI/ML technologies can be used to perform predictive maintenance, which involves predicting when equipment is likely to fail and scheduling maintenance accordingly. For example, AI/ML algorithms can analyze historical maintenance data, equipment usage patterns, and other factors to predict when a piece of equipment is likely to fail. This level of foresight allows OEMs to schedule maintenance at the most convenient times, minimizing disruption to production schedules and reducing maintenance costs.
- Automated Maintenance Scheduling: AI/ML technologies can also be used to automate the maintenance scheduling process, reducing the need for manual intervention and minimizing the risk of human error. For example, AI/ML algorithms can automatically schedule maintenance based on equipment usage patterns, production schedules, and other factors, ensuring that maintenance is performed at the most appropriate times.
- Cost Reduction: By optimizing maintenance processes, AI/ML technologies can help OEMs achieve significant cost reductions, particularly in areas such as labor, parts, and equipment replacement. For example, AI/ML algorithms can identify opportunities to extend the life of equipment or reduce the frequency of maintenance, reducing overall maintenance costs.
4. Efficient Supplier Collaboration (40-45%)
Effective collaboration with suppliers is essential for successful inventory management. Traditional methods of supplier collaboration often rely on manual processes and limited data sharing, which can lead to inefficiencies, delays, and increased operational costs. AI/ML technologies offer a more sophisticated approach to supplier collaboration, enabling OEMs to achieve greater efficiency and cost savings.
- Real-Time Data Sharing: AI/ML technologies enable real-time data sharing between OEMs and their suppliers, improving visibility and collaboration across the supply chain. For example, AI/ML algorithms can provide suppliers with real-time visibility into inventory levels, production schedules, and demand forecasts, enabling them to plan more effectively and respond more quickly to changing market conditions.
- Supplier Performance Monitoring: AI/ML technologies can also be used to monitor supplier performance in real-time, identifying potential issues before they lead to operational disruptions. For example, AI/ML algorithms can analyze data from supplier performance metrics, such as on-time delivery rates, quality levels, and lead times, to identify trends and patterns that may indicate potential issues. This level of monitoring allows OEMs to take corrective action before a disruption occurs, improving overall supply chain efficiency.
- Automated Supplier Management: AI/ML technologies can also be used to automate supplier management processes, reducing the need for manual intervention and minimizing the risk of human error. For example, AI/ML algorithms can automatically update supplier contracts, manage supplier performance metrics, and handle supplier communications, ensuring that supplier relationships are managed efficiently and effectively.
- Cost Reduction: By optimizing supplier collaboration, AI/ML technologies can help OEMs achieve significant cost reductions, particularly in areas such as procurement, transportation, and inventory holding. For example, AI/ML algorithms can identify opportunities to reduce lead times, optimize transportation routes, and minimize inventory holding costs, reducing overall supply chain costs.
5. Automated Replenishment (35-45%)
Automated replenishment is a critical component of effective inventory management, particularly for OEMs that rely on just-in-time (JIT) inventory systems. Traditional methods of replenishment often rely on manual processes and limited data sharing, which can lead to inefficiencies, delays, and increased operational costs. AI/ML technologies offer a more sophisticated approach to replenishment, enabling OEMs to achieve greater efficiency and cost savings.
- Real-Time Inventory Monitoring: AI/ML technologies enable real-time inventory monitoring, providing OEMs with accurate and up-to-date snapshots of their inventory levels. For example, AI/ML algorithms can track the movement of goods within a warehouse, monitor stock levels across multiple locations, and identify discrepancies between physical and recorded inventory.
- Automated Replenishment Triggers: AI/ML technologies can also be used to automate replenishment triggers, reducing the need for manual intervention and minimizing the risk of stockouts or overstocking. For example, AI/ML algorithms can automatically trigger replenishment orders when inventory levels fall below a certain threshold, ensuring that stock levels are always maintained at optimal levels.
- Optimized Replenishment Schedules: AI/ML technologies can also be used to optimize replenishment schedules, reducing the need for manual intervention and minimizing the risk of human error. For example, AI/ML algorithms can automatically schedule replenishment orders based on inventory levels, demand forecasts, and other factors, ensuring that replenishment is performed at the most appropriate times.
- Cost Reduction: By optimizing replenishment processes, AI/ML technologies can help OEMs achieve significant cost reductions, particularly in areas such as procurement, transportation, and inventory holding. For example, AI/ML algorithms can identify opportunities to reduce lead times, optimize transportation routes, and minimize inventory holding costs, reducing overall supply chain costs.
Why Partnering with AQe Digital?
Direct Access to Top Executives: At AQe Digital, our CEO, CXO, and CTO are actively involved at every stage of the process. Clients have direct access to these senior leaders throughout the project. This hands-on involvement ensures high standards of quality, timely delivery, and exceptional service from start to finish.
Client-Centric Co-Creation: We work closely with clients throughout the project to create solutions that perfectly match their goals and needs. This collaborative approach helps us innovate and tailor our services to meet specific requirements.
Agility and Flexibility: We focus on being agile and scalable, allowing us to quickly adapt to changing data landscapes and business needs. Unlike rigid solutions, AQe Digital’s approach ensures we respond swiftly to market changes and operational demands.
Hyper-Personalized Engagement: We don’t offer a one-size-fits-all solution. Instead, we tailor our services to each client’s unique needs and preferences, providing a personalized experience that goes beyond expectations.
Conclusion
AI/ML technologies offer a transformative approach to inventory management, enabling OEMs to achieve significant improvements in efficiency and cost savings. By integrating AI/ML into their inventory management practices, OEMs can improve inventory tracking, optimize demand forecasting, implement proactive maintenance, enhance supplier collaboration, and automate replenishment processes. With these capabilities, OEMs can streamline their inventory management by 30-35%, reduce operational costs, and gain a competitive advantage in the market.
Get in touch with us for a detailed consultation on addressing your inventory management challenges. Discover how our AI/ML-driven data services can streamline your OEM’s inventory management by up to 35%. We’re here to help!
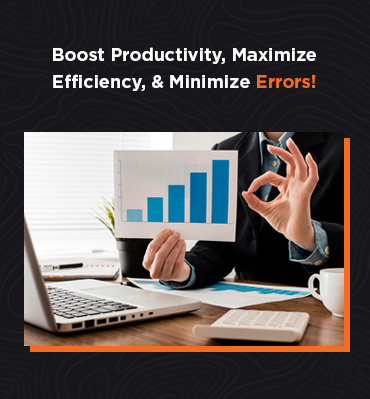